projects
Wearable brain-to-human communication
Jul 2022
Every 90 minutes, someone is diagnosed with Amyotrophic Lateral Sclerosis (ALS), and someone passes away from it 1.
Amyotrophic Lateral Sclerosis (ALS) is a relentlessly progressive disorder; muscle deterioration is slow and, currently, absolute. During the later stages, complete muscle failure is common. Current treatments (such as riluzole 2) only slow the progression, which can mean a longer time in the worst stages of the disease.
Muscle-based computer interfaces provide a stop-gap measure once speech is impossible - known as a locked-in state (LIS) - often providing a binary signal that can be used for basic communication (estimated prevalence of locked-in state: ~1 in 100,000 3).
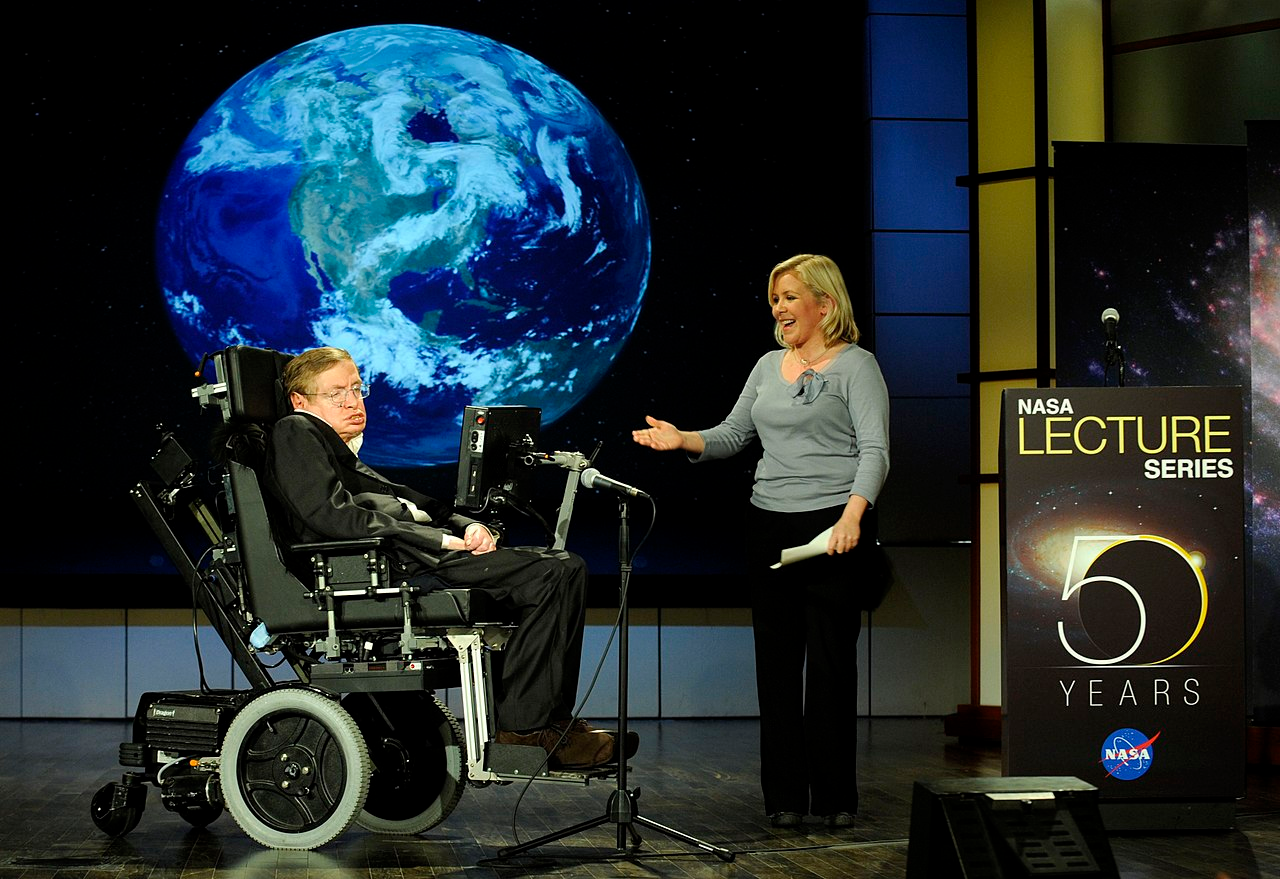
Stephen Hawking at NASA’s 50th anniversary in 2008. By 2008, he was limited to a binary signal operated via a “cheek switch”, even this simple signal allowed him to write a book and communicate. (NASA/Paul Alers Public Domain)
However, the usability of muscle-based communication is often fatiguing or unreliable, and worse, temporary. At the inevitable point of total muscle failure - a complete locked-in state (CLIS) - individuals lose the ability to communicate in any way.
Loss of communication occurs after the need for artificial respiration to live. 42% of those who risk losing the ability to be in touch with others say they would prefer to die rather than be unable to communicate 4; communication loss is consistently cited as a primary reason for refusing care 5.
Throughout this process the brain remains largely unaffected, as does, in most cases, the ability to think, imagine and feel emotions. Brain-computer interfaces (BCIs) can provide a communication channel that can endure through all stages of this disease, and restore a degree of agency when all other methods fail. Even the simplest BCI-based communication has a high impact on the perceived quality of life 6.
The feasibility of BCIs for communication is established with acceptable performance for a wide variety of applications:
- Spelling devices 7
- Finger control 8
- Web browsing 9
- Emotion detection 10
- Control for virtual reality environments 11.
Yet, despite over 22,000 BCI research studies 12, few acknowledge and adapt to the restrictions of real-life settings. For example, Wolpaw et al. 13 found only 3 case studies that describe independent home use of a BCI, and Craik et al. 14 surveyed that “more than a quarter of the studies (26 of 90)” removed artifacts manually making them unusable for real-time.
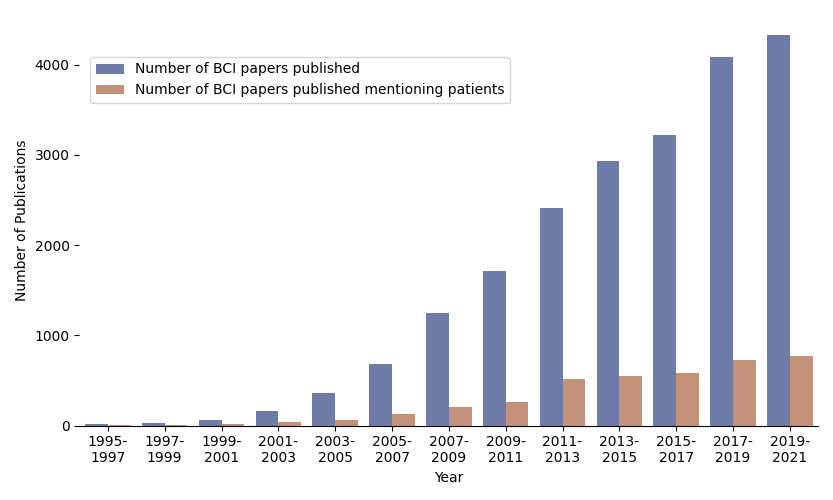
BCI-related publications in Scopus (the largest database of peer-reviewed literature), with a naive comparison between the presence of “patient”/“als” in the title, abstract, or keywords. While BCIs have a strong growth year on year, patient-based studies have shown a more linear progression. This aligns with the prediction made by Kübler 15
To scale BCIs for widespread application there is a need to reconsider laboratory-controlled setups, which don’t reflect use in the home, where there is no expert supervisor on hand to assist.
As we discovered, this is a step that is sorely needed. Existing algorithms with high accuracy rates (over 70% forming a baseline for usability) in literature cannot be applied without significant performance penalties.
Beyond the Medical
Many of the applications, the infrastructure and algorithms needed for real-world usage can be applied and expanded to consumer contexts, and more extreme environments. The benefits of usability ( from the wearable form-factor and wireless flexibility) are universal.
The Economist report on the AttentivU project, with a particular focus on low-gravity usage.
Contributions
RealityEXG
To measure the impact of these assumptions, we establish a platform for realistic brain signal data collection. This generated our Real-Life Dataset (“RealityEXG”), a substantial dataset of 67.2 hours across 16 participants, 9 devices, 488 disjointed sessions, and 122 days. This data is purposefully poor: it has a high proportion of artifacts; it is collected on commercially viable devices (with limited data collection); uses Bluetooth for transmission.
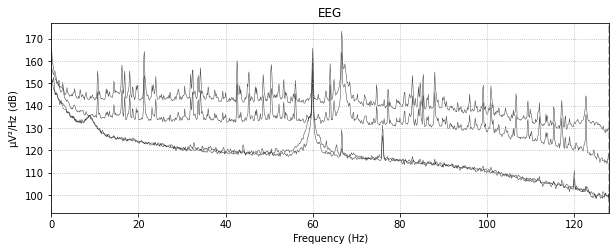
A Power Spectral Densities (PSD) of a given run with clear broken channels present, distinctive by the flat PSD curve suggesting white-noise corruption. Notably, there is a distinctive spike that suggests eyes were closed in this segment.
Mental Mathematics Model
Having established a mechanism to collect challenging data. We then proposed a novel BCI for communication using unstructured mental mathematics to enable basic communication without sensory stimuli. Our model runs in real-time and simply requires a mobile device and consumer-level headband/glasses. By removing the carefully applied EEG cap we can expand usage beyond the lab, and outside the norm.
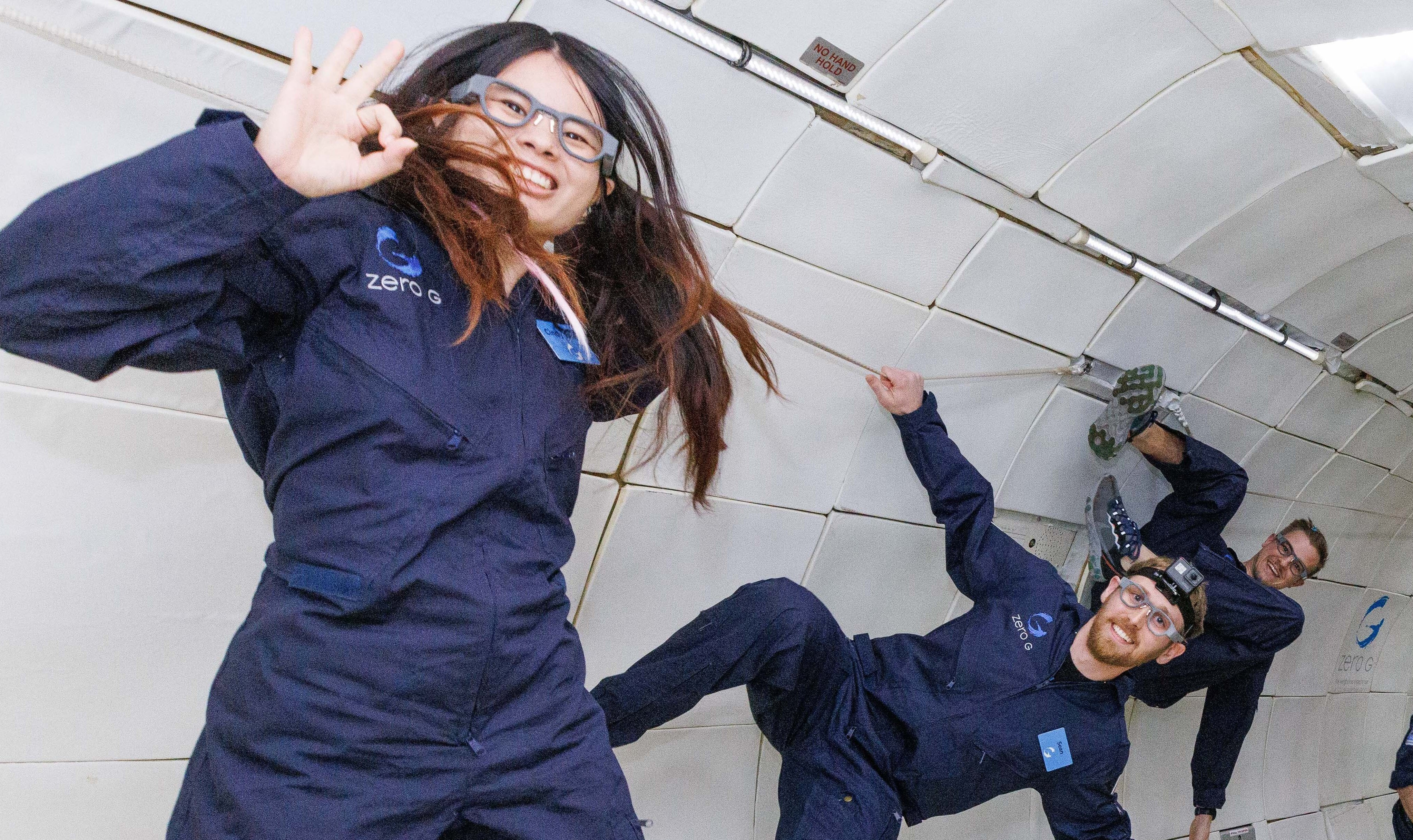
Exemplary usage of 3 pairs of AttentivU glasses in a zero-gravity environment. Data is recorded simultaneously and streamed to a linked mobile device.
Unstructured mental mathematics is simply the reaction to being told “perform mental maths”; we do not provide equations to solve, which would require a working visual system. This lack of structure makes detection both challenging, and very personal but also widely applicable. One person may focus heavily on numerical imagination and recall, while another may focus on working memory multiplying large numbers.
Despite this, the produced BCI can be used immediately by any new user without individual training, although accuracy jumps with tuning (76% accuracy on RealityEXG). By contrast, existing implementations 16 give over 90% accuracies on single-session lab environment inference, but fail to exceed chance when applied to noisy, consumer-grade datasets.
Further details on the initial ‘BrainSwitch’ model can be found in the full thesis text, or you can ask me directly to see the latest work on this topic.
Potential
Despite wishing we could achieve fluid, speech-level communication with our study participants, that goal is not yet realizable. Ethically, however, we must strive to achieve non-invasive, affordable BCIs; and despite our desire for perfection, a device that brings back an urgently needed function, that supplies a modicum of agency and dignity, or provides a back-up to existing approaches, is possibly a good start.
Providing individuals with control over basic but humanizing activities (requesting eye drops, asking for food, or starting music) allows for some dignity and agency against a disease that is constantly eroding their humanity.
Challenges
With the goal of fluid, speech-level communication in mind, I leave my list of challenges and limitations that should be overcome:
- Challenge 1: Efficiency. The potential for improvement in decoding algorithms is evident as we still require a large comparison window (>15s) to detect a mental state, resulting in a non-ideal information transfer rate of .
- Challenge 2: Supervised Algorithms do not transfer to noisy, low-channel count environments. The application of successfully supervised machine learning algorithms applied to computer vision and natural language processing tasks has proven ineffective. We attribute this to the poor signal-to-noise ratio common to EEG that is absent in other use cases. We suspect that appropriate inference requires further modifications to existing architectures to exploit the assumed second-order stationary nature of signals and the de-biasing for recently processed data. We do expect supervised strategies to exceed bio-physiological inspired strategies in the long term but are yet to determine the appropriate combination of feature extraction and network architecture to realize this performance.
- Challenge 3: Lack of free-text entry. One option would be to add a grid-speller similar to P300 to allow for the binary signal to create free-form text by submitting a character by encoding a row and column selection similar to the “Query” task for choosing environment requests. However, the speed of such an approach would be challenging, instead applying multi-modal contrastive algorithms, that relate EXG data to text, may allow for language-aware text models that combat the slow processing speed of signals.
- Challenge 4: Mental initiation. Currently, a question has to be asked before a response can be given. Allowing for proactive communication, where, for example, the mental math task can be used to turn on the “BrainSwitch” model would be far more effective. We have started to approach this using change detection of the ratios (rapid changes in ratio suggest the start of a mental task) but further work is needed.
- Challenge 5: Public distribution of the application suite Currently the applications are distributed to participants via “TestFlight”/manual installation. To allow for scaling up we would need to release the app to the App Store, move inference to occur locally, and allow data collection to be opted-in rather than mandatory.
- Challenge 6: Small electrode count causing low signal-to-noise-ratio. A large contributor to the difficulty of the decoding process was the lack of additional channels to perform noise reduction on alternative form factors that have either additional electrodes or allow for different EEG channel montages (e.g. such as over the head with a headphone form factor) that may provide channel locations particularly conducive to different mental states.
- Challenge 7: Lack of training data. Despite small sample sizes being commonplace in EEG studies, it likely doesn’t aid the supervised learning algorithms. Collecting more data would help and the application, as well as the infrastructure, is designed to support that; but additionally, the use of pre-text tasks on a large, unlabelled corpus of EEG data such as the TUH EEG Corpus which contains over a year of data, should help in overcoming the limitations. Examples considered include jigsaw puzzles in time-series segments (un-shuffling should learn time-series trends), transformation reversal (to denoise more effectively), and prediction of spectral decomposition (to learn spectral relationships).
Findings
The end-to-end system is ready for home-use, and provides basic communication functionality achieving several goals:
- Effective decoding. Our decoding algorithm, with subject-dependent tuning, reached % cross-session, cross-day accuracy, exceeding the % requirement for BCI accuracy. Subject independence in real-time settings was also possible at a performance penalty achieving % accuracy.
- Satisfying training times. The desired accuracy can be reached without user training or calibration time.
- Usable for long durations. The dry electrodes, long battery life (5-7 hours), comfortable form factor, and the use of a mobile phone base station allow for BCI to remain passive, waiting to be used when needed.
- Satisfying setup times. The combination of form factor, mobile app, and infrastructure all combine to allow for a yes/no question to be asked from no-setup within 60 seconds, rather than minutes 13 for an industry-standard home-use BCI.
Footnotes
-
The epidemiology of neuromuscular disorders: A comprehensive overview of the literature.
Deenen JC, Horlings CG, Verschuuren JJ, Verbeek AL, Engelen BG van Journal of neuromuscular diseases. 2015;2(1):73–85. ↩ -
A controlled trial of riluzole in amyotrophic lateral sclerosis.
Bensimon G, Lacomblez L, Meininger V, Group AS New England Journal of Medicine. 1994;330(9):585–91. ↩ -
Estimated prevalence of the target population for brain-computer interface neurotechnology in the netherlands.
Pels EG, Aarnoutse EJ, Ramsey NF, Vansteensel MJ Neurorehabilitation and neural repair. 2017;31(7):677–85. ↩ -
Patients with amyotrophic lateral sclerosis receiving long-term mechanical ventilation: Advance care planning and outcomes.
Moss AH, Oppenheimer EA, Casey P, Cazzolli PA, Roos RP, Stocking CB, et al Chest. 1996;110(1):249–55. ↩ -
Requests for euthanasia: Origin of suffering in ALS, heart failure, and cancer patients.
Maessen M, Veldink JH, Berg LH van den, Schouten HJ, Wal G van der, OnwuteakaPhilipsen BD Journal of neurology. 2010;257(7):1192–8. ↩ -
Speech therapy and communication device: Impact on quality of life and mood in patients with amyotrophic lateral sclerosis.
Körner S, Siniawski M, Kollewe K, Rath KJ, Krampfl K, Zapf A, et al Amyotrophic Lateral Sclerosis and Frontotemporal Degeneration. 2013;14(1):20–5. ↩ -
The mental prosthesis: Assessing the speed of a P300-based brain-computer interface.
Donchin E, Spencer KM, Wijesinghe R IEEE transactions on rehabilitation engineering. 2000;8(2):174–9. ↩ -
Decoding of individuated finger movements using surface electromyography.
Tenore FV, Ramos A, Fahmy A, Acharya S, Etienne-Cummings R, Thakor NV IEEE transactions on biomedical engineering. 2008;56(5):1427–34. ↩ -
Design and implementation of a P300-based brain-computer interface for controlling an internet browser.
Mugler EM, Ruf CA, Halder S, Bensch M, Kubler A IEEE Transactions on Neural Systems and Rehabilitation Engineering. 2010;18(6):599–609. ↩ -
Estimation of valence of emotion using two frontal EEG channels.
Wu S, Xu X, Shu L, Hu B In: 2017 IEEE international conference on bioinformatics and biomedicine (BIBM). IEEE; 2017: p. 1127–30. ↩ -
Openvibe: An open-source software platform to design, test, and use brain–computer interfaces in real and virtual environments.
Renard Y, Lotte F, Gibert G, Congedo M, Maby E, Delannoy V, et al Presence. 2010;19(1):35–53. ↩ -
Query from scorpus.com in 2022. ↩
-
Independent home use of a brain-computer interface by people with amyotrophic lateral sclerosis.
Wolpaw JR, Bedlack RS, Reda DJ, Ringer RJ, Banks PG, Vaughan TM, et al Neurology. 2018;91(3):e258–67. ↩ ↩2 -
Deep learning for electroencephalogram (EEG) classification tasks: A review.
Craik A, He Y, Contreras-Vidal JL Journal of neural engineering. 2019;16(3):031001. ↩ -
Hearing the needs of clinical users.
Kübler A, Nijboer F, Kleih S. Handbook of clinical neurology. 2020;168:353–68. ↩ -
Deep models for engagement assessment with scarce label information
Li F, Zhang G, Wang W, Xu R, Schnell T, Wen J, et al. IEEE Transactions on Human-Machine Systems. 2016;47(4):598–605. ↩